|
ACCESS THE FULL ARTICLE
No SPIE Account? Create one
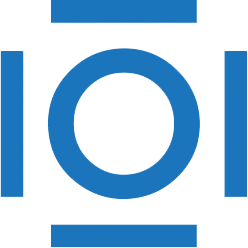
CITATIONS
Cited by 4 scholarly publications.
Particle filters
Particles
System identification
Digital filtering
Filtering (signal processing)
Nonlinear filtering
Monte Carlo methods