|
ACCESS THE FULL ARTICLE
No SPIE Account? Create one
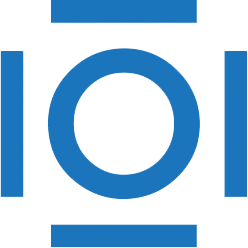
CITATIONS
Cited by 1 scholarly publication.
Neurons
Neural networks
Brain mapping
Aluminum
Electronics engineering
Optical character recognition
Optical signal processing