|
ACCESS THE FULL ARTICLE
No SPIE Account? Create one
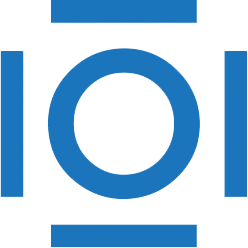
CITATIONS
Cited by 1 scholarly publication.
Neural networks
Speckle pattern
Holography
Finite element methods
Artificial neural networks
Nondestructive evaluation
Holograms