|
ACCESS THE FULL ARTICLE
No SPIE Account? Create one
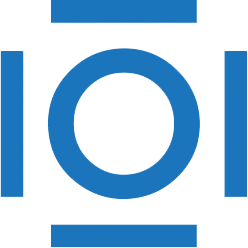
CITATIONS
Cited by 15 scholarly publications.
Binary data
Data acquisition
Data mining
Detection and tracking algorithms
Fuzzy logic
Knowledge discovery
Computer engineering