|
ACCESS THE FULL ARTICLE
No SPIE Account? Create one
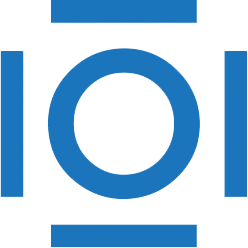
CITATIONS
Cited by 1 scholarly publication.
Tissues
Autoregressive models
Image segmentation
3D modeling
Error analysis
Magnetic resonance imaging
Statistical analysis