|
ACCESS THE FULL ARTICLE
No SPIE Account? Create one
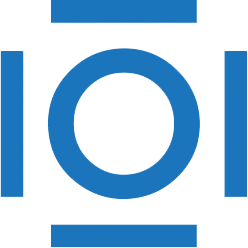
CITATIONS
Cited by 14 scholarly publications.
Associative arrays
Stochastic processes
Wavelets
Image processing
Statistical analysis
Algorithm development
Independent component analysis