|
ACCESS THE FULL ARTICLE
No SPIE Account? Create one
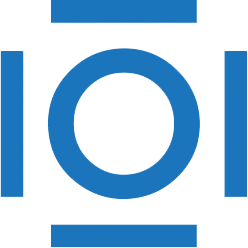
CITATIONS
Cited by 6 scholarly publications.
Feature extraction
Scattering
Synthetic aperture radar
Fourier transforms
Performance modeling
Image classification
Model-based design