|
ACCESS THE FULL ARTICLE
No SPIE Account? Create one
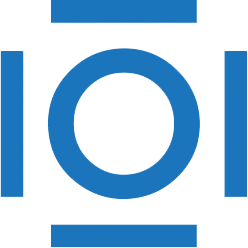
CITATIONS
Cited by 4 scholarly publications.
Binary data
Lithium
Neural networks
Analog electronics
Legal
Pattern recognition
Promethium