|
ACCESS THE FULL ARTICLE
No SPIE Account? Create one
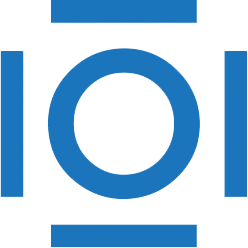
CITATIONS
Cited by 53 scholarly publications.
Wavelets
Expectation maximization algorithms
Wavelet transforms
Data modeling
Discrete wavelet transforms
Information technology
Image analysis