|
ACCESS THE FULL ARTICLE
No SPIE Account? Create one
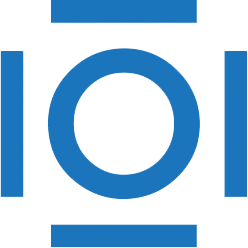
CITATIONS
Cited by 20 scholarly publications.
Signal to noise ratio
Sensors
Calibration
Data modeling
Reflectivity
Performance modeling
Systems modeling