|
ACCESS THE FULL ARTICLE
No SPIE Account? Create one
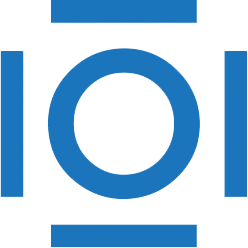
CITATIONS
Cited by 1 scholarly publication.
Target detection
Neural networks
Image processing
Infrared imaging
Radar
3D acquisition
Binary data