|
ACCESS THE FULL ARTICLE
No SPIE Account? Create one
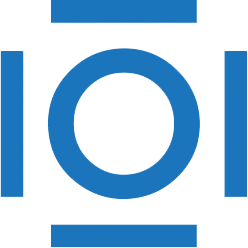
CITATIONS
Cited by 1 scholarly publication.
Feature extraction
Neural networks
Pattern recognition
Machine learning
Analog electronics
Brain mapping
Matrices