|
ACCESS THE FULL ARTICLE
No SPIE Account? Create one
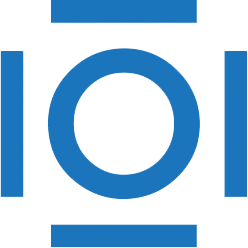
CITATIONS
Cited by 2 scholarly publications.
Data fusion
Sensors
Image fusion
Image classification
Remote sensing
L band
Probability theory