|
ACCESS THE FULL ARTICLE
No SPIE Account? Create one
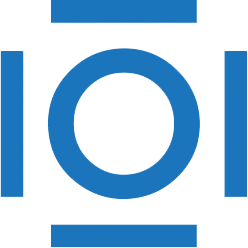
CITATIONS
Cited by 10 scholarly publications.
Binary data
Neural networks
Neurons
Artificial neural networks
Image classification
Classification systems
Computer engineering