|
ACCESS THE FULL ARTICLE
No SPIE Account? Create one
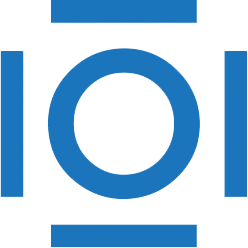
CITATIONS
Cited by 2 scholarly publications.
Visual communications
Distortion
Radon
Computer programming
Image processing
Image compression
Quantization