|
ACCESS THE FULL ARTICLE
No SPIE Account? Create one
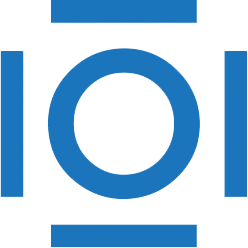
CITATIONS
Cited by 1 scholarly publication.
Radar
Neural networks
Extremely high frequency
Automatic target recognition
Error analysis
Artificial neural networks
Matrices