|
ACCESS THE FULL ARTICLE
No SPIE Account? Create one
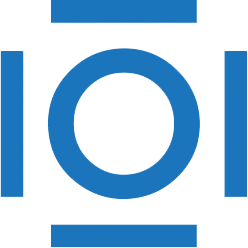
CITATIONS
Cited by 1 patent.
Performance modeling
Convolution
Image classification
Neural networks
Convolutional neural networks
Electrochemical etching
Image compression