|
ACCESS THE FULL ARTICLE
No SPIE Account? Create one
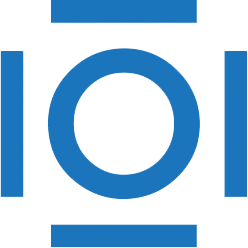
CITATIONS
Cited by 5 scholarly publications.
Lung
Chest imaging
Computer aided diagnosis and therapy
Computer aided design
Convolutional neural networks
Machine learning
Medical imaging