|
ACCESS THE FULL ARTICLE
No SPIE Account? Create one
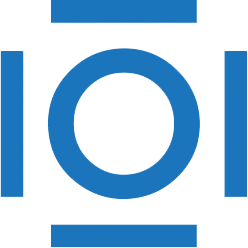
CITATIONS
Cited by 1 scholarly publication.
Signal to noise ratio
Time-frequency analysis
Neural networks
Convolution
Denoising
Fourier transforms