|
ACCESS THE FULL ARTICLE
No SPIE Account? Create one
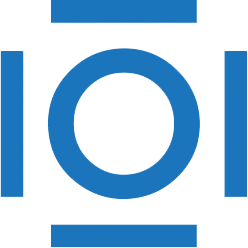
CITATIONS
Cited by 5 scholarly publications.
Comets
Neural networks
Machine learning
Data modeling
Error analysis
Network architectures
Analytical research