|
ACCESS THE FULL ARTICLE
No SPIE Account? Create one
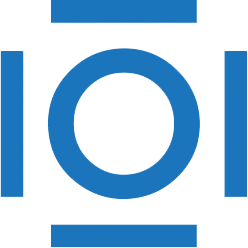
CITATIONS
Cited by 1 scholarly publication.
Data modeling
Neural networks
Computer simulations
Performance modeling
Convolution
Visualization
Image segmentation