|
ACCESS THE FULL ARTICLE
No SPIE Account? Create one
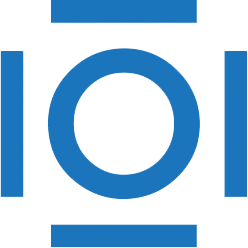
CITATIONS
Cited by 2 scholarly publications.
Image segmentation
Dendrites
Tomography
Materials science
X-rays
Aluminum
Convolutional neural networks