|
ACCESS THE FULL ARTICLE
No SPIE Account? Create one
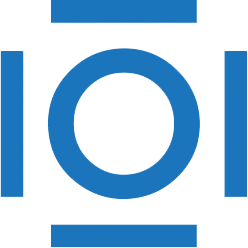
CITATIONS
Cited by 1 scholarly publication.
Resonators
Refractive index
Machine learning
Plasmonic waveguides
Waveguides
Sensors
Finite-difference time-domain method