|
ACCESS THE FULL ARTICLE
No SPIE Account? Create one
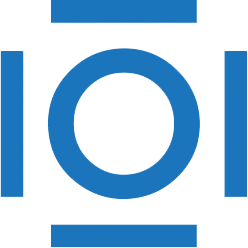
CITATIONS
Cited by 2 scholarly publications and 3 patents.
Image segmentation
Magnetic resonance imaging
Image filtering
Cardiovascular magnetic resonance imaging
Medical imaging
Convolutional neural networks
Imaging systems