|
ACCESS THE FULL ARTICLE
No SPIE Account? Create one
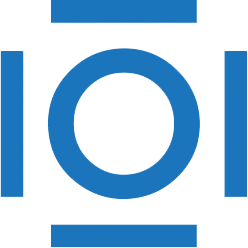
CITATIONS
Cited by 1 scholarly publication.
Video
Optical tracking
Video surveillance
Detection and tracking algorithms
Convolutional neural networks
Feature extraction
Network architectures