|
ACCESS THE FULL ARTICLE
No SPIE Account? Create one
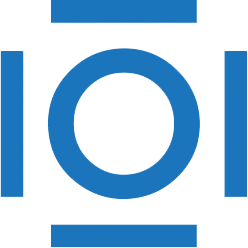
CITATIONS
Cited by 3 scholarly publications.
Target detection
Monte Carlo methods
Automatic tracking
Digital filtering
Process modeling
Electronic filtering
Data modeling