The sKPCA-AD has proven successful using unordered spectral pixel data, however, anomalies are often larger objects composed of many neighboring pixels. In this paper we show that fusing spatial information derived from a panchromatic image with spectral information from a hyper/multispectral image can increase the accuracy of the sKPCA-AD. We accomplish this by creating several joint spectral-spatial kernels that are then used by the sKPCA-AD to learn the underlying background model. We take into account the variability introduced by the random subsampling by showing averaged results and variance over several skeletons. We test our methods on two representative datasets and our results show improved performance with one of the proposed joint kernel methods. |
ACCESS THE FULL ARTICLE
No SPIE Account? Create one
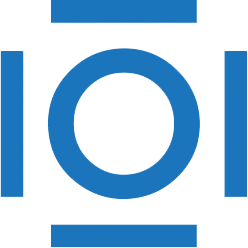
CITATIONS
Cited by 1 scholarly publication.
Data modeling
Image fusion
Hyperspectral imaging
Principal component analysis
Data fusion
Multispectral imaging
Detection and tracking algorithms