|
ACCESS THE FULL ARTICLE
No SPIE Account? Create one
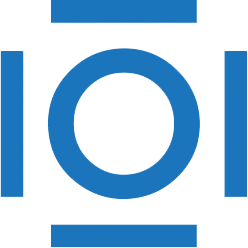
CITATIONS
Cited by 8 scholarly publications.
Photomasks
Optical proximity correction
SRAF
Optimization (mathematics)
Lithography
Image transmission
Image processing