|
ACCESS THE FULL ARTICLE
No SPIE Account? Create one
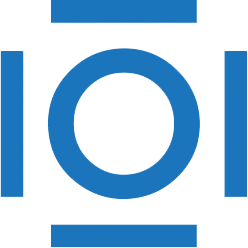
CITATIONS
Cited by 1 scholarly publication.
Data modeling
Nanoparticles
Liver
Plasma
Mathematical modeling
Tumors
Bayesian inference