The purpose of this paper is to introduce new features of the AAR-recognition approach (abbreviated as AAR-R from now on) of combining texture and intensity information into the recognition procedure, using the optimal spanning tree to achieve the optimal hierarchy for recognition to minimize recognition errors, and to illustrate recognition performance by using large-scale testing computed tomography (CT) data sets. The data sets pertain to 216 non-serial (planning) and 82 serial (re-planning) studies of head and neck (H&N) cancer patients undergoing radiation therapy, involving a total of ~2600 object samples. Texture property “maximum probability of occurrence” derived from the co-occurrence matrix was determined to be the best property and is utilized in conjunction with intensity properties in AAR-R. An optimal spanning tree is found in the complete graph whose nodes are individual objects, and then the tree is used as the hierarchy in recognition. Texture information combined with intensity can significantly reduce location error for glandrelated objects (parotid and submandibular glands). We also report recognition results by considering image quality, which is a novel concept. AAR-R with new features achieves a location error of less than 4 mm (~1.5 voxels in our studies) for good quality images for both serial and non-serial studies. |
ACCESS THE FULL ARTICLE
No SPIE Account? Create one
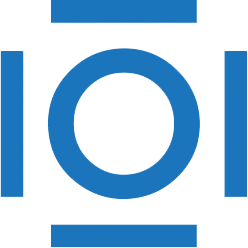
CITATIONS
Cited by 10 scholarly publications.
Image quality
Computed tomography
Data modeling
Neck
Image segmentation
Radiotherapy
Head