|
ACCESS THE FULL ARTICLE
No SPIE Account? Create one
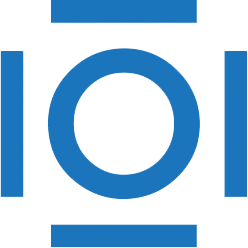
CITATIONS
Cited by 1 scholarly publication.
Heart
Lung
Sensors
Data modeling
3D modeling
Target detection
Convolution