|
ACCESS THE FULL ARTICLE
No SPIE Account? Create one
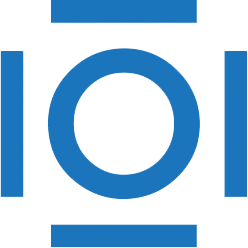
CITATIONS
Cited by 17 scholarly publications.
Digital breast tomosynthesis
Breast
Image quality
Mammography
Breast cancer
Image quality standards
Denoising