|
ACCESS THE FULL ARTICLE
No SPIE Account? Create one
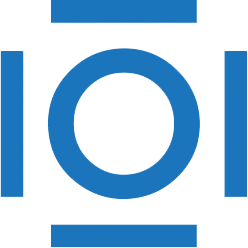
CITATIONS
Cited by 5 scholarly publications.
Robots
Computer intrusion detection
Information security
Network security
Neural networks
Robotic systems
Databases