|
ACCESS THE FULL ARTICLE
No SPIE Account? Create one
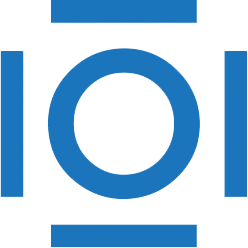
CITATIONS
Cited by 13 scholarly publications.
Image segmentation
Image registration
Brain
Head
Magnetic resonance imaging
Detection and tracking algorithms
Image processing algorithms and systems