Background: Ultrasound (US)-guided fine needle aspiration (FNA) cytology is the gold standard for the evaluation of thyroid nodules. However, up to 30% of FNA results are indeterminate, requiring further testing. In this study, we present a machine-learning analysis of indeterminate thyroid nodules on ultrasound with the aim to improve cancer diagnosis. Methods: Ultrasound images were collected from two institutions and labeled according to their FNA (F) and surgical pathology (S) diagnoses [malignant (M), benign (B), and indeterminate (I)]. Subgroup breakdown (FS) included: 90 BB, 83 IB, 70 MM, and 59 IM thyroid nodules. Margins of thyroid nodules were manually annotated, and computerized radiomic texture analysis was conducted within tumor contours. Initial investigation was conducted using five-fold cross-validation paradigm with a two-class Bayesian artificial neural networks classifier, including stepwise feature selection. Testing was conducted on an independent set and compared with a commercial molecular testing platform. Performance was evaluated using receiver operating characteristic analysis in the task of distinguishing between malignant and benign nodules. Results: About 1052 ultrasound images from 302 thyroid nodules were used for radiomic feature extraction and analysis. On the training/validation set comprising 263 nodules, five-fold cross-validation yielded area under curves (AUCs) of 0.75 [Standard Error (SE) = 0.04; P < 0.001] and 0.67 (SE = 0.05; P = 0.0012) for the classification tasks of MM versus BB, and IM versus IB, respectively. On an independent test set of 19 IM/IB cases, the algorithm for distinguishing indeterminate nodules yielded an AUC value of 0.88 (SE = 0.09; P < 0.001), which was higher than the AUC of a commercially available molecular testing platform (AUC = 0.81, SE = 0.11; P < 0.005). Conclusion: Machine learning of computer-extracted texture features on gray-scale ultrasound images showed promising results classifying indeterminate thyroid nodules according to their surgical pathology. |
ACCESS THE FULL ARTICLE
No SPIE Account? Create one
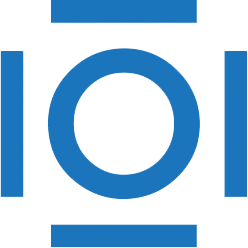
CITATIONS
Cited by 9 scholarly publications.
Fine needle aspiration
Ultrasonography
Cancer
Surgery
Medicine
Pathology
Cell biology