|
ACCESS THE FULL ARTICLE
No SPIE Account? Create one
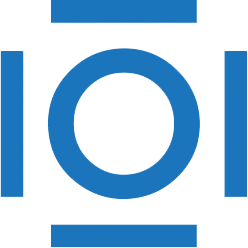
CITATIONS
Cited by 17 scholarly publications.
Image segmentation
Convolutional neural networks
Performance modeling
Data modeling
Breast
Breast cancer
Sensors