ACCESS THE FULL ARTICLE
No SPIE Account? Create one
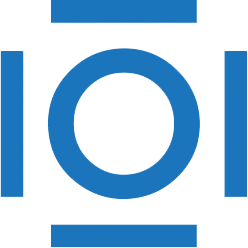
CITATIONS
Cited by 21 scholarly publications.
Image segmentation
Polyps
Medical imaging
Tumors
Brain
Data modeling
Performance modeling