|
ACCESS THE FULL ARTICLE
No SPIE Account? Create one
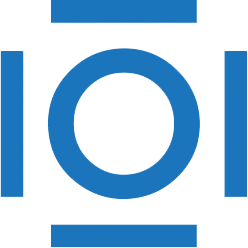
CITATIONS
Cited by 2 scholarly publications.
Image segmentation
Tumors
Liver
Computed tomography
Tissues
Medical imaging
Surgery