|
ACCESS THE FULL ARTICLE
No SPIE Account? Create one
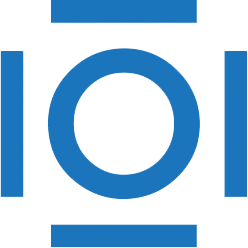
CITATIONS
Cited by 3 scholarly publications.
Feature extraction
Breast
Data modeling
Magnetic resonance imaging
Binary data
Feature selection
Breast cancer