|
ACCESS THE FULL ARTICLE
No SPIE Account? Create one
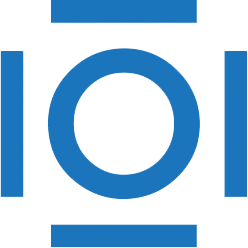
CITATIONS
Cited by 1 scholarly publication.
Tumors
Brain
Magnetic resonance imaging
Diffusion tensor imaging
Machine learning
Neuroimaging
Tissues