|
ACCESS THE FULL ARTICLE
No SPIE Account? Create one
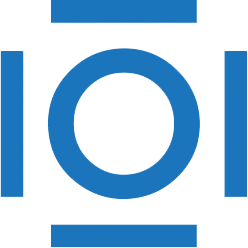
CITATIONS
Cited by 3 scholarly publications.
Land mines
Mining
Target detection
Polarization
Sensors
Statistical modeling
Data modeling