|
ACCESS THE FULL ARTICLE
No SPIE Account? Create one
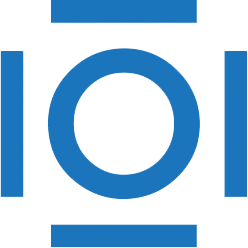
CITATIONS
Cited by 5 scholarly publications.
Liver
Image segmentation
Computed tomography
Signal attenuation
Convolution
Binary data
Tumors