|
ACCESS THE FULL ARTICLE
No SPIE Account? Create one
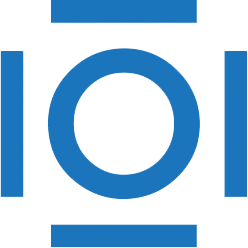
CITATIONS
Cited by 3 scholarly publications.
Biopsy
Cancer
Performance modeling
Fine needle aspiration
Ultrasonography
Data modeling
Classification systems