|
ACCESS THE FULL ARTICLE
No SPIE Account? Create one
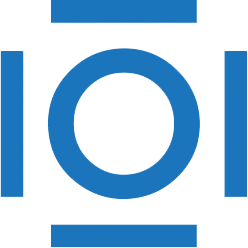
CITATIONS
Cited by 6 scholarly publications.
Heart
Pathology
Ultrasonography
Medical imaging
Artificial neural networks
Acoustics
Convolutional neural networks