|
ACCESS THE FULL ARTICLE
No SPIE Account? Create one
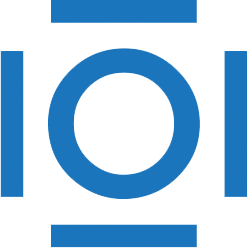
CITATIONS
Cited by 1 scholarly publication.
Data modeling
Databases
Mouth
Performance modeling
Facial recognition systems
Human-computer interaction
Image classification